Lowering the entry threshold for Neural Vector Search by applying Similarity Learning
Kacper Łukawski • Location: TUECHTIG • Back to Haystack EU 2022
Neural search is the hottest trend in the area of search engines. It is not debatable. Training a neural network is, in turn, thought to require a lot of annotated data and may struggle in some specific domains if we decide to use some pretrained models without any further fine-tuning. Similarity learning addresses most of the possible issues and allows training your models in hours or days, not months, with much-lowered data requirements, also in a rapidly changing environment. This talk will reveal how to design an end-to-end pipeline with some Open Source tools and describe some common pitfalls and the ways to avoid them.
Download the Slides Watch the Video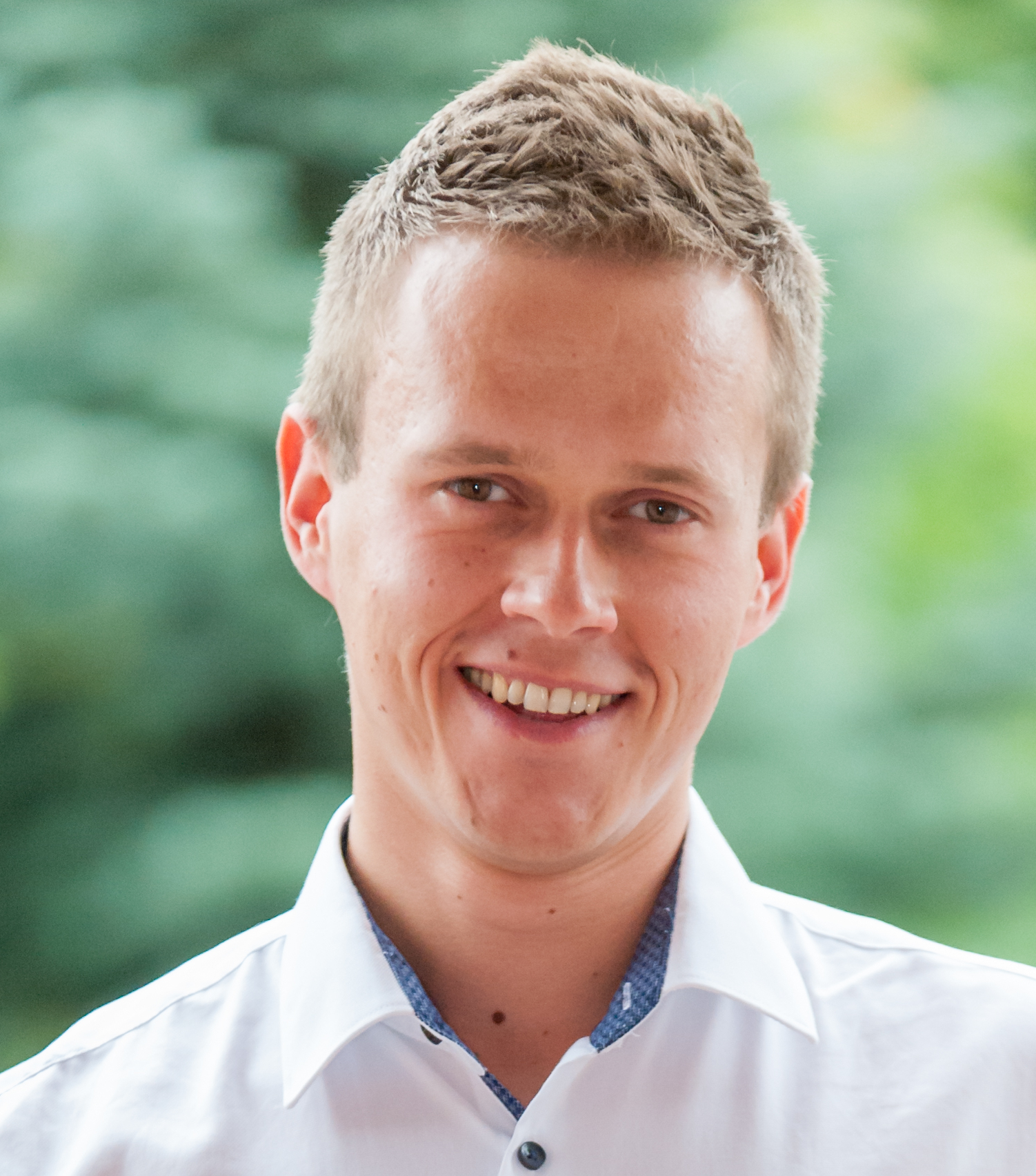
Kacper Łukawski
QdrantKacper Łukawski is a Developer Advocate at Qdrant - an open-source neural search engine. His broad experience is mostly related to data engineering, machine learning, and software design. He has been actively contributing to the discussion on Artificial Intelligence by conducting lectures and workshops locally and internationally. Recently he’s been exploring the world of similarity learning and vector search.