Big Vector Search - The Billion-Scale Approximate Nearest Neighbor Challenge
George Williams • Location: Theater 7 • Back to Haystack 2022
Despite the broad range of algorithms for Approximate Nearest Neighbor vector search, most empirical evaluations of algorithms have focused on smaller datasets, typically of 1 million points. However, deploying recent advances in embedding based techniques for search, recommendation and ranking at scale require ANNS indices at billion, trillion or larger scale. Barring a few recent papers, there is limited consensus on which algorithms are effective at this scale vis-`a-vis their hardware cost. We recently completed the first Billion-Scale Approximate Nearest Neighbor Challenge (sponsored by NeurIPS2021), which compared ANNS algorithms at billion-scale by hardware cost, accuracy, and performance on 6 billion scale datasets, most of them recently introduce to the community. We set up an open source evaluation for both standardized and specialized hardware. In this talk, we will discuss the new datasets and how we compared relative performance of the algorithms.
Download the Slides Watch the Video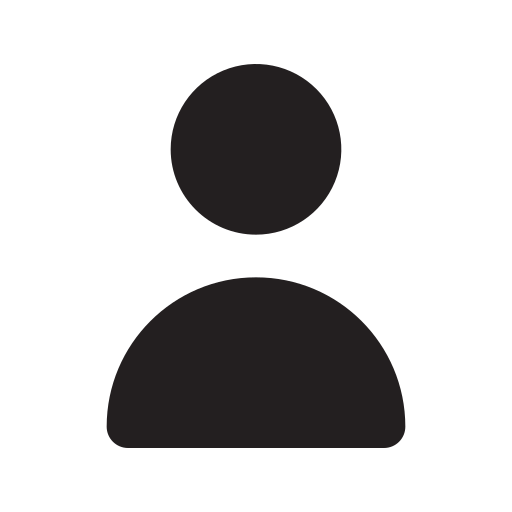
George Williams
Smile IdentitySpeaker biography coming soon!